Smarter, Adaptive Robots
Machine learning is transforming robotics by enabling control systems that can learn and adapt in real-time.
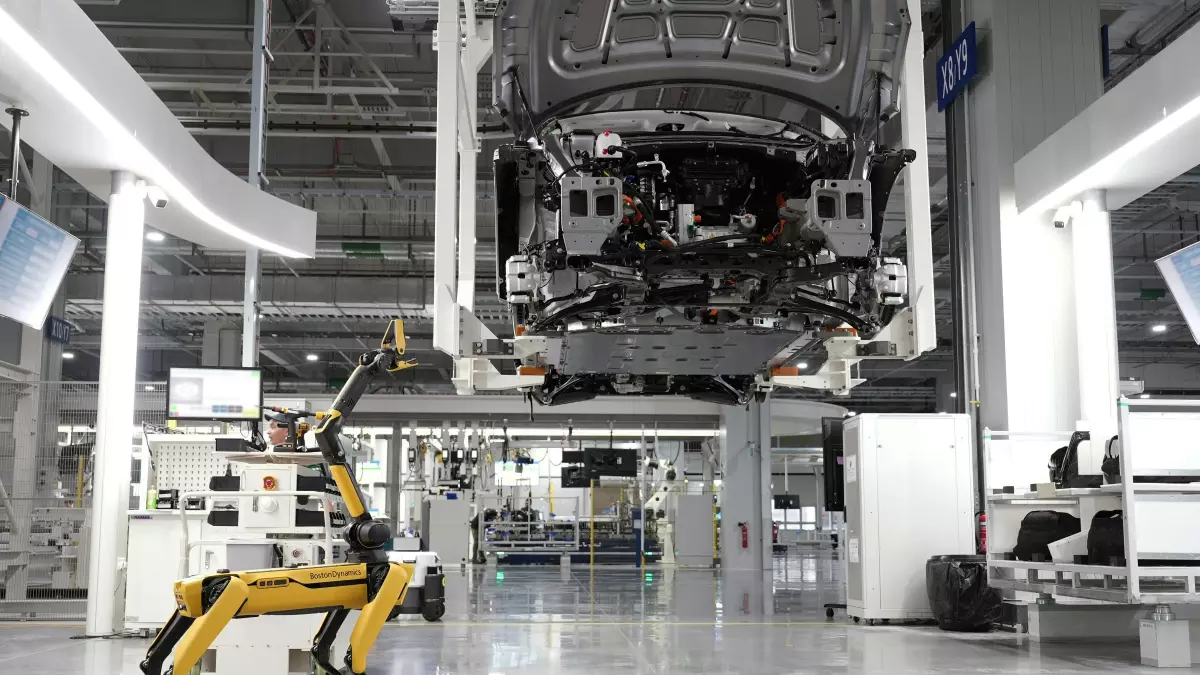
By Sophia Rossi
“The real problem is not whether machines think but whether men do.” – B.F. Skinner. This quote, from the famous psychologist, speaks volumes about the current state of robotics. We’ve reached a point where machines, particularly robots, are not just following pre-programmed instructions but are learning from their environment. And the driving force behind this? Machine learning.
Machine learning (ML) is a subset of artificial intelligence (AI) that allows systems to learn from data, identify patterns, and make decisions with minimal human intervention. In robotics, ML is revolutionizing how control systems operate, making robots smarter, more adaptive, and capable of handling complex, dynamic environments. But how exactly does this work? Let’s dive into the technical side of things.
Machine Learning in Control Systems: The Basics
At the heart of every robot is a control system. This system is responsible for managing the robot’s movements, decision-making, and interactions with the environment. Traditionally, control systems relied on predefined algorithms and rules. However, with machine learning, control systems can now evolve based on the data they receive.
For example, instead of programming a robot to avoid obstacles in a specific way, machine learning allows the robot to learn from its encounters with obstacles. Over time, the robot refines its approach, improving its ability to navigate complex environments. This is particularly useful in autonomous robots, where the environment is unpredictable, and pre-programmed responses may not always be effective.
How Machine Learning Enhances Adaptability
One of the biggest advantages of integrating machine learning into robotics control systems is adaptability. Robots equipped with ML algorithms can adjust their behavior based on real-time data. This means they can handle new situations without needing to be reprogrammed.
Take, for example, a warehouse robot tasked with sorting packages. Initially, it may struggle with packages of unusual shapes or sizes. However, through machine learning, the robot can learn from its mistakes and improve its sorting accuracy over time. This adaptability is crucial in industries like manufacturing, logistics, and even healthcare, where robots need to perform tasks in constantly changing environments.
Reinforcement Learning: A Game-Changer
One of the most exciting areas of machine learning in robotics is reinforcement learning (RL). In RL, robots learn by trial and error, receiving feedback from their actions. Think of it as a robot playing a video game – it tries different strategies, learns from its mistakes, and eventually masters the game.
In a robotics context, reinforcement learning allows robots to optimize their actions to achieve specific goals. For instance, a robot arm in a factory might use RL to figure out the most efficient way to assemble a product. Over time, the robot becomes faster and more precise, reducing errors and increasing productivity.
Challenges and Limitations
Of course, integrating machine learning into robotics control systems is not without its challenges. One of the biggest hurdles is the need for vast amounts of data. For a robot to learn effectively, it needs access to a wide range of experiences. This can be difficult to achieve in controlled environments where the robot’s interactions are limited.
Additionally, machine learning algorithms require significant computational power. While advances in hardware are helping to mitigate this issue, it remains a challenge for smaller, more compact robots that may not have the processing capacity to run complex ML models.
The Future of Robotics Control Systems
Despite these challenges, the future of robotics control systems is undeniably tied to machine learning. As algorithms become more sophisticated and hardware continues to improve, we can expect robots to become even more autonomous, adaptive, and intelligent.
Imagine a future where robots not only perform tasks but also learn to optimize those tasks on the fly. Whether it’s a robot surgeon learning to perform more precise incisions or a drone adapting to changing weather conditions, the possibilities are endless.
Machine learning is pushing the boundaries of what robots can do, and we’re just scratching the surface. As the technology continues to evolve, we’ll see robots that are not only tools but also partners in innovation, capable of learning and growing alongside us.