Learning Machines
Robots used to be like those kids in school who could memorize everything but didn’t really understand it. They could follow instructions to the letter, but if something unexpected happened, they were lost. Enter robot learning systems, the game-changer that’s turning robots from rigid rule-followers into adaptable, problem-solving machines.
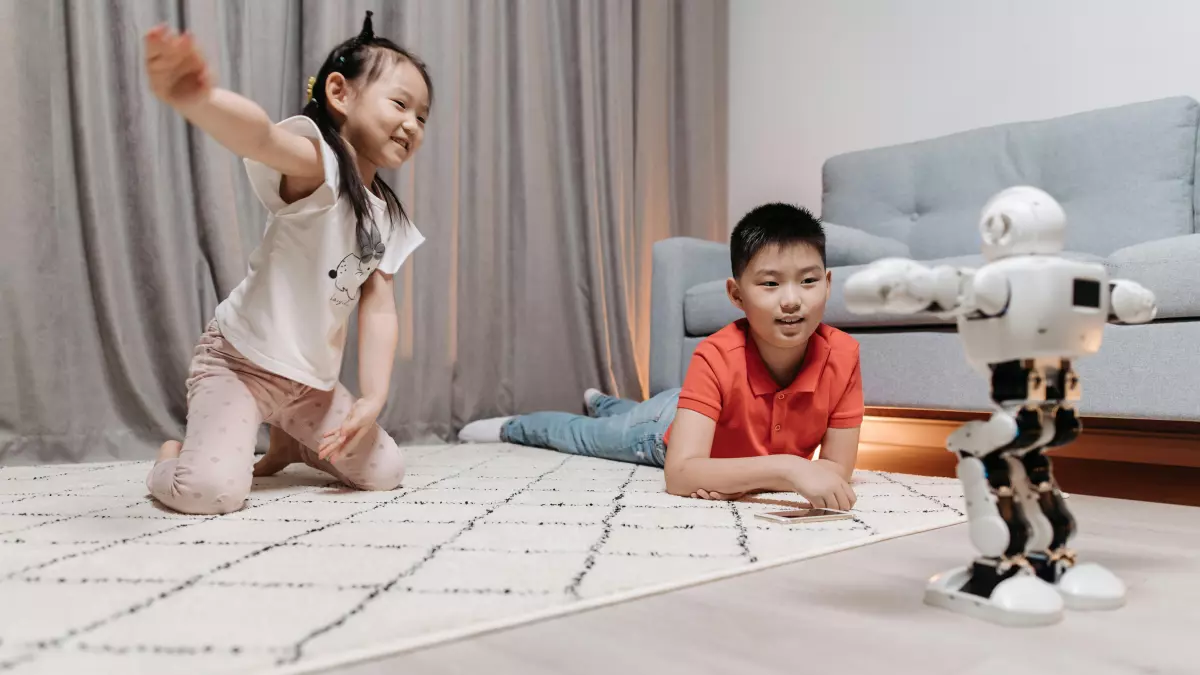
By Nina Schmidt
Let’s compare two types of robots: one that relies on pre-programmed instructions and another that learns from its environment. The first robot is like a GPS that only knows one route—if there’s a roadblock, it’s stuck. The second robot, however, is like a GPS that learns new routes as it goes, adapting to traffic, road closures, and even your driving habits. That’s the magic of robot learning systems.
At its core, robot learning is about giving machines the ability to improve their performance over time. Traditional robots execute tasks based on fixed programming, while learning robots adapt their behavior based on experience. This shift is transforming industries, from manufacturing to healthcare, where robots are now able to handle more complex, unpredictable tasks.
What Exactly Is Robot Learning?
Robot learning is a subset of artificial intelligence (AI) that focuses on teaching robots to learn from data, experiences, or interactions with their environment. Think of it as the difference between a robot that can only follow a recipe and one that can experiment in the kitchen, tweaking ingredients to improve the dish.
There are several approaches to robot learning, but the two most common are supervised learning and reinforcement learning. In supervised learning, robots are trained using labeled data, meaning they’re given examples of correct behavior and learn to replicate it. In reinforcement learning, robots learn through trial and error, receiving feedback (like rewards or penalties) based on their actions. It’s like teaching a dog to sit by giving it treats when it gets it right.
Hardware and Software: The Dynamic Duo
Robot learning systems rely on a combination of hardware and software to function. On the hardware side, sensors and actuators play a crucial role. Sensors allow robots to perceive their environment, while actuators enable them to interact with it. But the real magic happens in the software—the algorithms that process sensor data and make decisions.
One of the most exciting developments in robot learning is the use of neural networks, which mimic the human brain’s structure. These networks allow robots to process vast amounts of data and make decisions based on patterns they’ve learned. For example, a robot equipped with a neural network might learn to recognize objects in its environment, improving its ability to navigate and interact with the world.
Why Robot Learning Matters
So, why does all this matter? Because robot learning systems are the key to making robots more autonomous, flexible, and useful. In industries like manufacturing, robots that can learn from their mistakes can adapt to new tasks without needing to be reprogrammed. In healthcare, robots that learn from patient interactions can provide more personalized care.
But it’s not just about making robots smarter—it’s about making them safer, too. Robots that can learn from their environment are better equipped to avoid accidents and handle unexpected situations. For example, a robot in a warehouse might learn to navigate around obstacles or adjust its speed to avoid collisions with human workers.
The Challenges of Robot Learning
Of course, robot learning isn’t without its challenges. One of the biggest hurdles is the sheer amount of data required to train robots effectively. Just like humans, robots need a lot of practice to get good at something. This means collecting and processing massive amounts of data, which can be time-consuming and expensive.
Another challenge is ensuring that robots learn the right lessons. In reinforcement learning, for example, robots might develop unintended behaviors if they’re rewarded for the wrong actions. Imagine a robot vacuum that learns to push dirt under the rug because it gets rewarded for having a clean floor!
The Future of Robot Learning
Despite these challenges, the future of robot learning looks incredibly promising. As AI and machine learning technologies continue to advance, we can expect robots to become even more capable of learning and adapting. In the not-too-distant future, we might see robots that can teach themselves entirely new skills, like a factory robot that learns to assemble a new product without any human intervention.
In fact, some researchers are already working on robots that can learn from watching humans. These robots use a technique called imitation learning, where they observe human actions and try to replicate them. This could lead to robots that can learn new tasks simply by watching a human demonstrate them, making it easier than ever to train robots for complex jobs.
So, the next time you see a robot in action, remember: it might not just be following orders—it could be learning, adapting, and getting smarter with every move.
And here’s a fun fact to leave you with: according to a recent study, robots that use reinforcement learning can improve their performance by up to 50% compared to traditional robots. That’s a huge leap toward a future where robots are not just tools, but true collaborators.