Data Wrangling Unleashed
Did you know that data scientists spend up to 80% of their time wrangling data before they even get to the fun part—building models?
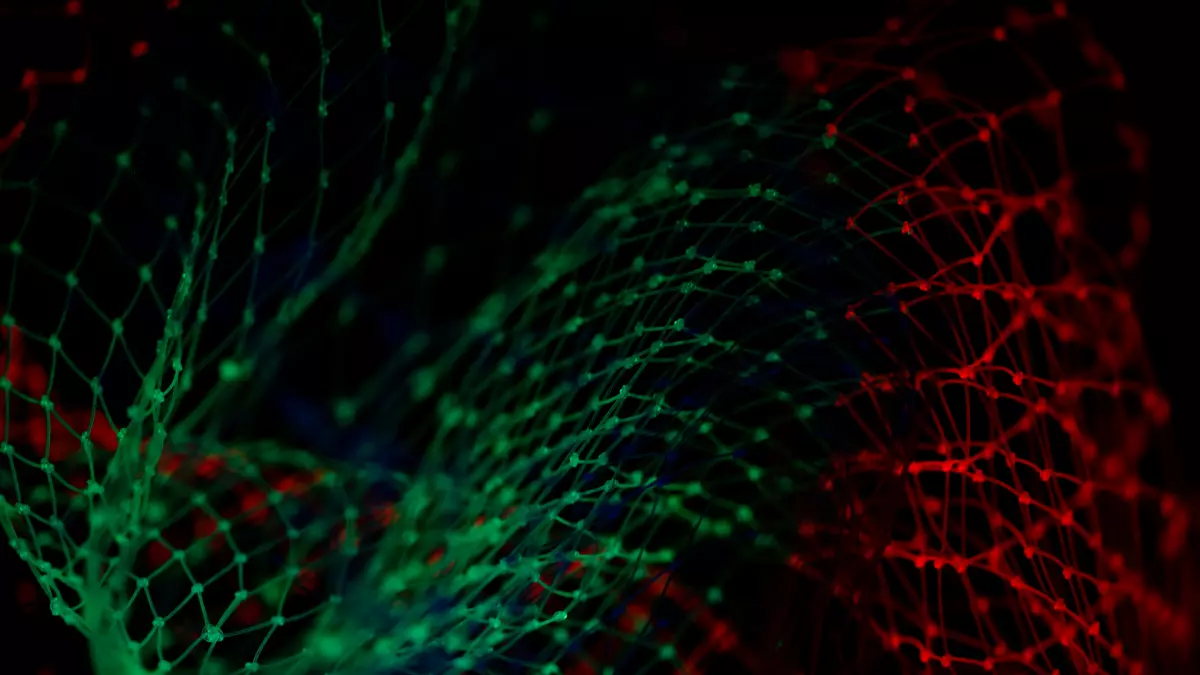
By James Sullivan
Yep, that’s right. Back in the day, data wrangling was a tedious, manual process that felt more like untangling a ball of yarn than anything remotely high-tech. But fast forward to today, and AI is stepping in to take the reins. Data wrangling, also known as data munging, is the process of cleaning, structuring, and enriching raw data into a more digestible format for analysis. It’s the unsung hero of the data science world, and AI is quietly revolutionizing it.
So, how did we get here? Let’s rewind for a second. In the early days of data science, wrangling was a manual process that involved a lot of human intervention. You had to clean up messy datasets, fill in missing values, and transform data into a usable format. It was time-consuming and error-prone. But as data grew in volume and complexity, the need for automation became clear. Enter AI.
AI has brought a new level of efficiency to data wrangling. Machine learning algorithms can now automatically detect patterns in data, identify outliers, and even suggest transformations. This means less time spent on grunt work and more time focusing on the actual analysis. AI-driven tools can handle everything from filling in missing values to normalizing data, and they’re getting smarter by the day.
One of the most exciting developments in AI-powered data wrangling is the use of Natural Language Processing (NLP). With NLP, AI can understand and process unstructured data like text, making it easier to extract valuable insights from sources like social media, customer reviews, and even emails. This is a game-changer for industries that rely heavily on unstructured data, such as marketing and customer service.
But it’s not just about cleaning and transforming data. AI is also helping with data enrichment. By integrating external datasets, AI can add context and depth to your data, making it more valuable for analysis. For example, AI can pull in weather data, economic indicators, or even social trends to give your dataset a richer, more complete picture.
So, what’s next for AI and data wrangling? Well, we’re already seeing the rise of self-service AI tools that allow non-technical users to wrangle data without needing a data science degree. These tools use AI to guide users through the wrangling process, making it more accessible to everyone. This democratization of data wrangling is going to be huge, especially as more businesses look to leverage data for decision-making.
In the future, we can expect AI to take on an even bigger role in data wrangling. As AI models become more sophisticated, they’ll be able to handle more complex data types and perform more advanced transformations. We might even see AI-driven tools that can automatically generate insights from raw data, eliminating the need for manual analysis altogether.
So, while data wrangling may not be the most glamorous part of data science, it’s definitely one of the most important. And with AI stepping in to streamline the process, it’s only going to get better from here.