Predictive Power Unleashed
Imagine AI as a crystal ball, peering into the future of your data with uncanny accuracy. But unlike mystics, AI relies on math, logic, and raw computational power to make predictions that can reshape industries.
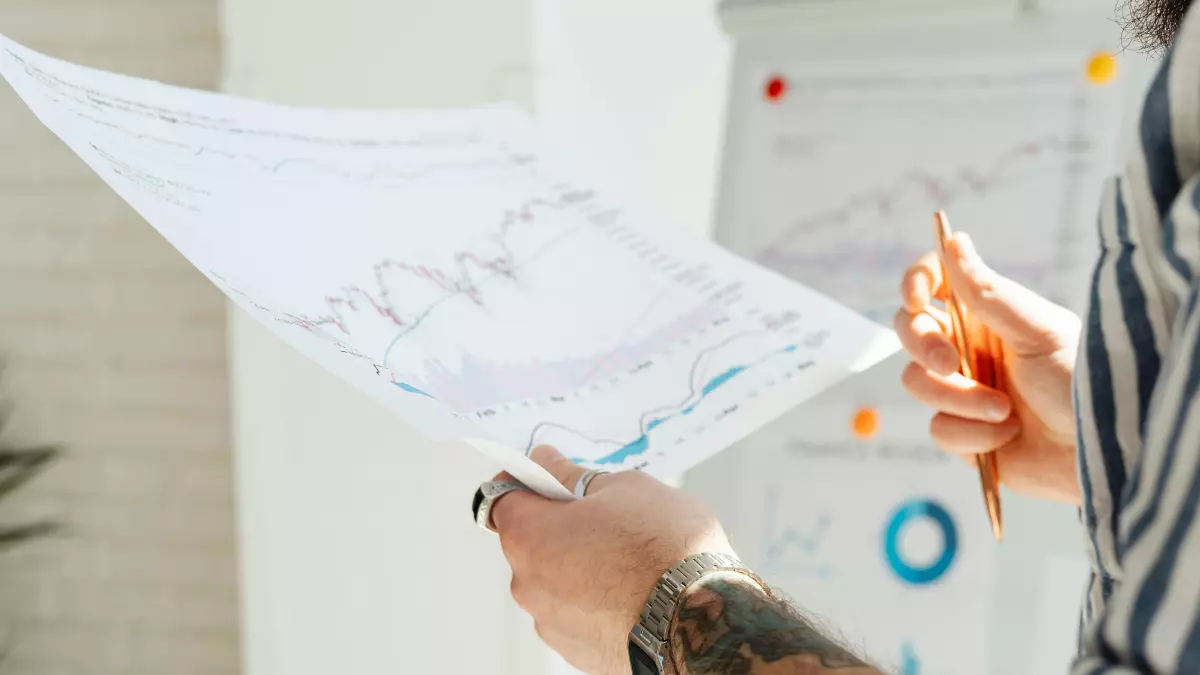
By Hiroshi Tanaka
In a world drowning in data, the ability to forecast trends, behaviors, and outcomes is like having a superpower. From predicting stock market shifts to anticipating customer preferences, data forecasting is the backbone of decision-making in the modern era. But traditional forecasting methods often fall short—they're slow, rigid, and prone to human error. Enter AI, the game-changer that’s rewriting the rules of predictive analytics.
AI-driven data forecasting isn’t just about crunching numbers faster; it’s about uncovering patterns and insights that were previously invisible. Thanks to machine learning algorithms, neural networks, and advanced statistical models, AI can analyze vast datasets, adapt to new information, and refine its predictions in real-time. Sounds like science fiction? It’s not—it’s happening right now.
How AI Makes Forecasting Smarter
Traditional forecasting methods rely heavily on historical data and predefined models. While this approach works for stable environments, it struggles with dynamic, fast-changing conditions. AI, on the other hand, thrives in complexity. Here’s how:
- Dynamic Learning: Machine learning models can continuously learn and adapt as new data streams in, making them far more accurate than static models.
- Pattern Recognition: AI excels at identifying subtle patterns and correlations that humans might overlook, even in noisy or incomplete datasets.
- Scenario Analysis: AI can simulate multiple "what-if" scenarios, helping businesses prepare for various outcomes and make informed decisions.
For instance, consider a retail company trying to forecast product demand. Traditional methods might use last year’s sales data as a baseline. AI, however, can incorporate a multitude of variables—weather patterns, social media trends, economic indicators—and provide a nuanced, real-time forecast. The result? Better inventory management, reduced waste, and happier customers.
The Algorithms Behind the Magic
At the heart of AI-driven forecasting are sophisticated algorithms. Let’s break down a few of the key players:
- Time Series Models: These include ARIMA (AutoRegressive Integrated Moving Average) and LSTM (Long Short-Term Memory) networks, which are particularly effective for sequential data like stock prices or weather patterns.
- Regression Analysis: A staple of predictive analytics, regression models are enhanced by AI to handle nonlinear relationships and high-dimensional data.
- Ensemble Methods: Techniques like Random Forests and Gradient Boosting combine multiple models to improve accuracy and robustness.
Each algorithm has its strengths and weaknesses, and the choice depends on the specific forecasting task. The beauty of AI is its ability to integrate these methods, creating hybrid models that deliver the best of all worlds.
Real-World Applications
AI-driven forecasting is making waves across industries. Here are a few examples:
- Finance: Predicting stock market trends, credit risks, and fraud detection.
- Healthcare: Forecasting disease outbreaks, patient admissions, and treatment outcomes.
- Supply Chain: Anticipating demand fluctuations, optimizing logistics, and managing risks.
- Energy: Predicting energy consumption, optimizing grid operations, and integrating renewable sources.
These applications are not just theoretical; they’re being implemented by companies and organizations worldwide, driving efficiency, reducing costs, and improving outcomes.
The Challenges Ahead
Of course, AI in data forecasting isn’t without its challenges. Data quality remains a critical issue—garbage in, garbage out, as the saying goes. Ensuring that datasets are clean, comprehensive, and unbiased is essential for accurate predictions. Additionally, the "black box" nature of some AI models can make it difficult to interpret how decisions are made, raising ethical and regulatory concerns.
Then there’s the issue of scalability. While AI models can handle large datasets, they require significant computational resources, which can be a barrier for smaller organizations. However, advancements in cloud computing and edge AI are gradually leveling the playing field.
The Future of Forecasting
As AI continues to evolve, so will its capabilities in data forecasting. Expect to see more integration with IoT devices, enabling real-time data collection and analysis. Imagine a smart city where traffic patterns, energy usage, and public safety are all optimized through AI-driven forecasts. The possibilities are endless.
But perhaps the most exciting prospect is the democratization of AI. With user-friendly tools and platforms, even non-experts will be able to harness the power of AI for forecasting, making this technology accessible to everyone.
So, the next time you hear someone talk about AI in data forecasting, remember: it’s not just about predicting the future—it’s about shaping it. And in a world where uncertainty is the only constant, that’s a power worth having.