AI vs Traditional Data
Remember when you had to wait for your computer to process a simple Excel sheet, and it felt like watching paint dry? Well, times have changed, but not all data processing has caught up.
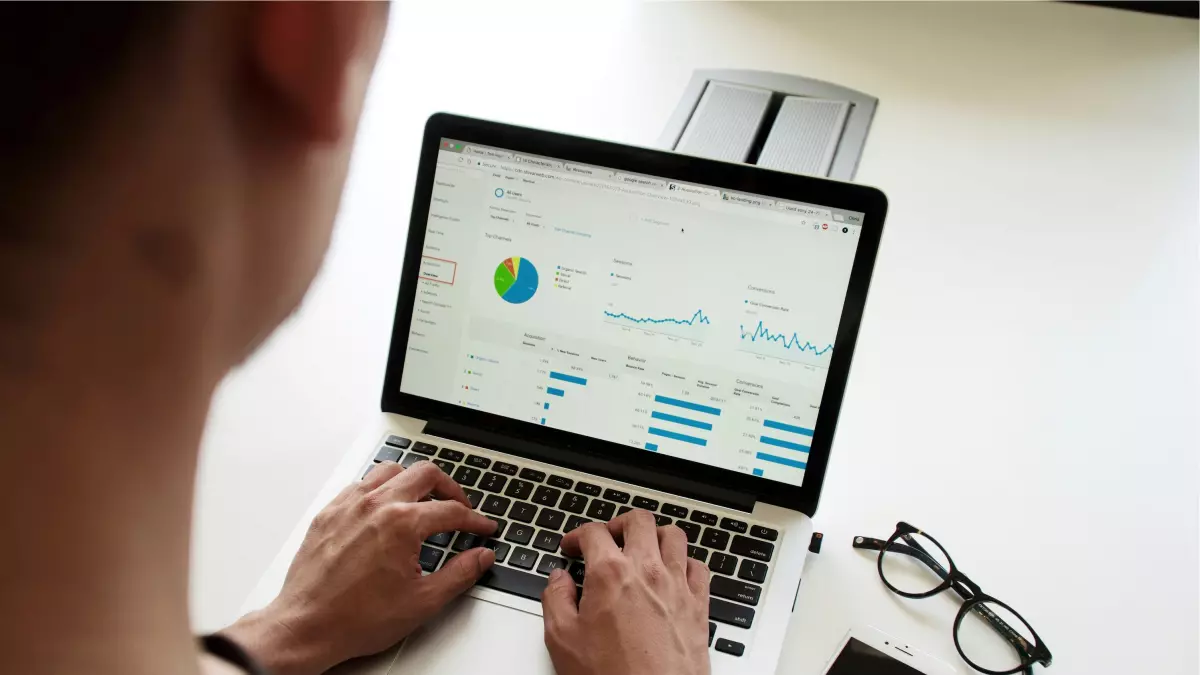
By Elena Petrova
Back in the day, traditional data processing was the only game in town. You’d throw your data into a system, and it would crunch numbers, spit out reports, and maybe even give you a headache or two. Fast forward to today, and we’ve got AI swooping in like a superhero, promising faster, smarter, and more efficient data processing. But is AI really the knight in shining armor we’ve been waiting for, or is traditional data processing still holding its ground?
Traditional Data Processing: The Old Reliable
Let’s not throw shade at traditional data processing just yet. It’s been around for decades, and for good reason. It’s reliable, predictable, and, well, it works. Traditional systems use predefined algorithms and rules to process data. Think of it like following a recipe—step-by-step, no surprises. You know exactly what you’re going to get.
Traditional data processing is great when you have structured data, like numbers in a spreadsheet or entries in a database. It’s also a solid choice when you need to process data in batches, like generating monthly reports or analyzing historical data. The downside? It can be slow, especially when dealing with large datasets. And let’s not even talk about unstructured data—traditional systems tend to throw a fit when faced with text, images, or videos.
AI-Driven Data Processing: The New Kid on the Block
Enter AI, the flashy new kid who promises to do everything faster, better, and with a lot more flair. AI-driven data processing doesn’t just follow a recipe—it learns from the data and adapts. It’s like a chef who can whip up a new dish based on what’s in the fridge, without needing a recipe at all.
AI excels at handling unstructured data, which is becoming more and more important in today’s data-driven world. Think social media posts, customer reviews, or even video footage—AI can process all of that and more. It’s also great for real-time data processing, making it a go-to for industries like finance, healthcare, and e-commerce, where decisions need to be made in the blink of an eye.
But here’s the catch: AI-driven data processing isn’t perfect. It requires a lot of data to learn from, and if that data is biased or incomplete, well, you’re going to get some pretty wonky results. Plus, AI systems can be complex to set up and maintain, requiring specialized skills and resources.
Speed vs Accuracy: The Eternal Tug-of-War
One of the biggest differences between AI and traditional data processing is speed. AI can process massive amounts of data in real-time, while traditional systems tend to work in batches. But speed isn’t everything—accuracy matters too. Traditional systems are often more accurate when dealing with structured data, while AI can sometimes struggle with precision, especially if it hasn’t been trained properly.
So, which one wins? Well, it depends on what you’re looking for. If you need to process large amounts of unstructured data quickly, AI is your best bet. But if you’re working with structured data and need rock-solid accuracy, traditional data processing might still be the way to go.
The Future: A Hybrid Approach?
Here’s a thought: why not use both? Many companies are starting to adopt a hybrid approach, combining the best of both worlds. AI can handle the heavy lifting when it comes to unstructured data and real-time processing, while traditional systems can take care of the more structured, batch-based tasks. It’s like having both a chef and a recipe book in your kitchen—sometimes you need creativity, and sometimes you just want to follow the steps.
At the end of the day, the choice between AI and traditional data processing comes down to your specific needs. Do you need speed, flexibility, and the ability to handle unstructured data? Or do you prioritize accuracy, reliability, and simplicity? The good news is, you don’t have to choose just one. With the right strategy, you can have the best of both worlds.
So, next time you’re faced with a data processing challenge, don’t just reach for the old reliable or the shiny new toy. Take a step back, assess your needs, and consider a hybrid approach. You might just find that the perfect solution is a little bit of both.